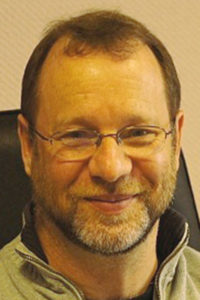
Imperial College, UK
Data assimilation and dynamic observers: from sparse measurements to flow information and control
In many simulations and experiments we are faced with the task of recovering full flow information from either reduced-order models or sparse (in space or time) measurement signals. The underlying mathematical concepts – data assimilation, Kalman filters and dynamic state estimation – are subject to many challenges, such as regularity and accuracy of the solution, robustness to environmental noise and appropriateness of standard tools.
We will review the principal concepts of data assimilation and dynamic observers and touch upon some of the difficulties in these approaches. A model problem will guide our efforts, and applications to channel flow, boundary layers, and Rayleigh-Benard convection will demonstrate our methodology. Various extensions and generalizations will also be discussed.
Short Biography
Peter Schmid currently holds a Chair Professorship of Applied Mathematics and Mathematical Physics at the Department of Mathematics of Imperial College London. Before joining the department in 2013, he held a position of research director with the French National Research Agency (CNRS) and a professorship (PCC) at the Ecole Polytechnique in France. Before then, he was a faculty member in Applied Mathematics at the University of Washington in Seattle, WA. He received his Ph.D. in Mathematics from M.I.T. and his Engineer’s Degree in Aerospace Engineering from the Technical University Munich. His research lies in the aera of computational fluid mechanics, with emphasis on stability theory, receptivity theory, flow control, model reduction and system identification. He is also interested in methods for quantitative flow analysis for numerical and experimental data.