Keynote Speakers
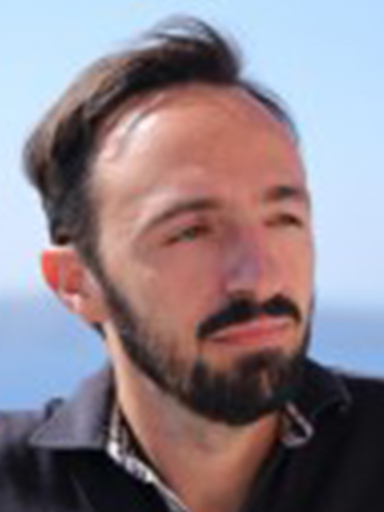
Professor Georgios N Yannakakis
Institute of Digital Games, Calamatta Building, University of Malta, Msida
Title: AI designing games for us... with (or without) us
Abstract: Can computational processes and machine-crafted artifacts be considered creative? When does this happen and who judges after all? What happens when we create together with a creative machine? Do we merely create together or can a machine truly foster our creativity as human creators? When does such co-creation foster the creativity of both humans and machines? In this talk I will address the above questions by positioning computer games as the ideal application domain for artificial intelligence for the unique features they offer. Advanced methods for autonomous game design, procedural content generation and AI-assisted game design will be showcased via a plethora of projects running currently at the Institute of Digital Games.
Short Biography: Georgios N. Yannakakis (yannakakis.net) is a Professor at the University of Malta (UoM) and the Director of the Institute of Digital Games. He has received the PhD degree in Informatics from the University of Edinburgh in 2006. Prior to joining the Institute of Digital Games, UoM, in 2012 he was an Associate Professor at the Center for Computer Games Research at the IT University of Copenhagen. He does research at the crossroads of artificial intelligence, computational creativity, affective computing, advanced game technology, and human-computer interaction. In particular, he pursues research concepts such as user experience modeling and procedural content generation for the design of personalized interactive systems for entertainment, education, training and health.
He has published over 200 journal and conference papers in the aforementioned fields and his work has been cited over 6700 times with an h-index of 44. His research has been supported by numerous national and European grants and has appeared in Science Magazine and New Scientist among other venues. He is currently an Associate Editor of the IEEE Transactions on Games and was an Associate Editor of the IEEE Transactions on Affective Computing between 2009 and 2017. He has been the General Chair of key conferences in the area of game artificial intelligence (IEEE CIG 2010) and games research (FDG 2013). He is the co-author of the Artificial Intelligence and Games textbook.
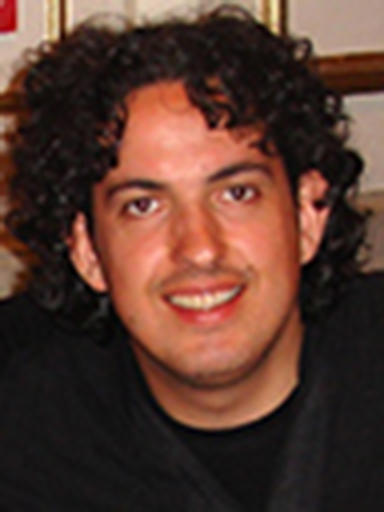
Professor Anastasios Tefas
Aristotle University of Thessaloniki, Department of Informatics, Greece
Title: "Deep Learning and Robotics: perception, control and innovations"
Abstract: This keynote speech will focus on deep learning methods and their use in robotics for increased perception, control and other innovative tasks. Deep learning emerged as one of the most promising research fields in artificial intelligence. The significant advancements that deep learning methods have brought out for large scale image classification tasks have generated a surge of excitement in applying the techniques to other problems in computer vision and more broadly into other disciplines of computer science, such as robotics. However, building deep learning algorithms for highly non-linear real-world problems such as those encountered in computer vision and robotics is non-trivial and requires substantial expertise. Unmanned Aerial Vehicles (drones) are among the robotic units that have substantial needs for autonomous control and perception due to their increasing use in several applications like transportation, inspection, surveillance and cinematography among others. Deep Convolutional Neural Networks (CNNs) are among the state-of-the-art techniques for Visual Information Analysis that can provide increased perception capabilities. CNNs can be used to perform several robotic perception tasks such as object detection and tracking, face detection and person identification, crowd detection for ensuring flight safety on drones, emergency landing point detection, etc. However, deploying such deep learning models on drones or other robotic units is not a straightforward task, since there are significant memory and model complexity constraints. To overcome these limitations several methodologies have been proposed: a)training small lightweight CNNs, b)using knowledge transfer techniques, such as neural-network distillation, layer hints and similarity embeddings, to reduce the size of CNNs and c) using neural region proposals for fast object detection and classification (faster R-CNN, YOLO, SSD).
Furthermore, gathering training data suitable for training the deep learning models is also a challenging task. Learning by using dataset augmentation techniques, such as hard negative and positive sample mining, can help to partially overcome this limitation, while allows us to further increase the performance of the trained models. Deep learning techniques can be also used for end-to-end drone control, allowing the deep model to control every aspect of the flight, from the visual information analysis to the drone and camera controls. Using multiple drones (multidrone setup) can increase the flexibility of drone cinematography. Such systems can be adaptive and evolving. Finally, there are several deep learning frameworks that can be used for deploying the aforementioned deep learning techniques on robotics (Tensorflow, Caffe, Theano, Darknet, etc).
Short Biography: Anastasios Tefas received the B.Sc. in informatics in 1997 and the Ph.D. degree in informatics in 2002, both from the Aristotle University of Thessaloniki, Greece. Since 2017 he has been an Associate Professor at the Department of Informatics, Aristotle University of Thessaloniki. From 2008 to 2017, he was a Lecturer, Assistant Professor at the same University. From 2006 to 2008, he was an Assistant Professor at the Department of Information Management, Technological Institute of Kavala. From 2003 to 2004, he was a temporary lecturer in the Department of Informatics, University of Thessaloniki. From 1997 to 2002, he was a researcher and teaching assistant in the Department of Informatics, University of Thessaloniki. Dr. Tefas participated in 12 research projects financed by national and European funds. He has co-authored 76 journal papers, 188 papers in international conferences and contributed 8 chapters to edited books in his area of expertise. Over 3900 citations have been recorded to his publications and his H-index is 33 according to Google scholar. His current research interests include computational intelligence, deep learning, pattern recognition, statistical machine learning, robotics, digital signal and image analysis and retrieval and computer vision.
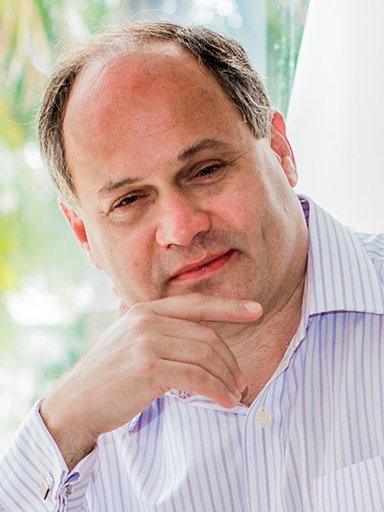
Professor Plamen Angelov
Lancaster University, United Kingdom
Title: Empirical Approach to Learning from Data (Streams): Fast and Interpretable Deep Learning
Abstract: We are witnessing an explosion of data (streams) being generated and growing exponentially. Nowadays we carry in our pockets Gigabytes of data in the form of USB flash memory sticks, smartphones, smartwatches etc. Extracting useful information and knowledge from these big data streams is of immense importance for the society, economy and science. Deep Learning quickly become a synonymous of a powerful method to enable items and processes with elements of AI in the sense that it makes possible human like performance in recognising images and speech. However, the currently used methods for deep learning which are based on neural networks (recurrent, belief, etc.) is opaque (not transparent), requires huge amount of training data and computing power (hours of training using GPUs), is offline and its online versions based on reinforcement learning has no proven convergence, does not guarantee same result for the same input (lacks repeatability).
The speaker recently introduced a new concept of empirical approach to machine learning and fuzzy sets and systems, had proven convergence for a class of such models and used the link between neural networks and fuzzy systems (neuro-fuzzy systems are known to have a duality from the radial basis functions (RBF) networks and fuzzy rule based models and having the key property of universal approximation proven for both).
In this talk he will present in a systematic way the basics of the newly introduced Empirical Approach to Machine Learning, Fuzzy Sets and Systems and its applications to problems like: anomaly detection, clustering, classification, prediction and control. The major advantages of this new paradigm is the liberation from the restrictive and often unrealistic assumptions and requirements concerning the nature of the data (random, deterministic, fuzzy), the need to formulate and assume a priori the type of distribution models, membership functions, the independence of the individual data observations, their large (theoretically infinite) number, etc. From a pragmatic point of view, this direct approach from data (streams) to complex, layered model representation is automated fully and leads to very efficient model structures. In addition, the proposed new concept learns in a way similar to the way people learn – it can start from a single example. The reason why the proposed new approach makes this possible is because it is prototype based and non-parametric.
Profile: Prof. Angelov (MEng 1989, PhD 1993, DSc 2015) is a Fellow of the IEEE, of the IET and of the HEA. He is Vice President of the International Neural Networks Society (INNS) for Conference and Governor of the Systems, Man and Cybernetics Society of the IEEE. He has 25+ years of professional experience in high level research and holds a Personal Chair in Intelligent Systems at Lancaster University, UK. He leads the Data Science group at the School of Computing and Communications which includes over 20 academics, researchers and PhD students. He has authored or co-authored 300 peer-reviewed publications in leading journals, peer-reviewed conference proceedings, 6 patents, two research monographs (by Wiley, 2012 and Springer, 2002) cited over 6700+ times with an h-index of 39 and i10-index of 116. His single most cited paper has 810 citations. He has an active research portfolio in the area of computational intelligence and machine learning and internationally recognised results into online and evolving learning and algorithms for knowledge extraction in the form of human-intelligible fuzzy rule-based systems. Prof. Angelov leads numerous projects (including several multimillion ones) funded by UK research councils, EU, industry, UK MoD. His research was recognised by 'The Engineer Innovation and Technology 2008 Special Award' and 'For outstanding Services' (2013) by IEEE and INNS. He is also the founding co-Editor-in-Chief of Springer's journal on Evolving Systems and Associate Editor of several leading international scientific journals, including IEEE Transactions on Fuzzy Systems (the IEEE Transactions with the highest impact factor) of the IEEE Transactions on Systems, Man and Cybernetics as well as of several other journals such as Applied Soft Computing, Fuzzy Sets and Systems, Soft Computing, etc. He gave over a dozen plenary and key note talks at high profile conferences. Prof. Angelov was General co-Chair of a number of high profile conferences including IJCNN2013, Dallas, TX; IJCNN2015, Killarney, Ireland; the inaugural INNS Conference on Big Data, San Francisco; the 2nd INNS Conference on Big Data, Thessaloniki, Greece and a series of annual IEEE Symposia on Evolving and Adaptive Intelligent Systems. Dr Angelov is the founding Chair of the Technical Committee on Evolving Intelligent Systems, SMC Society of the IEEE and was previously chairing the Standards Committee of the Computational Intelligent Society of the IEEE (2010-2012). He was also a member of International Program Committee of over 100 international conferences (primarily IEEE). More details can be found at www.lancs.ac.uk/staff/angelov.
Organized by
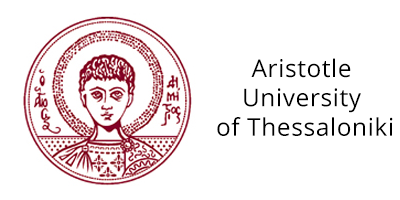
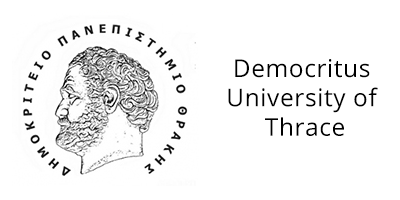
Sponsored by
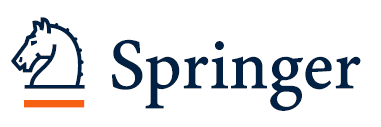
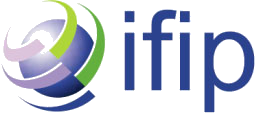